PyTorch = Numpy + AutoGrad
라는 개인적인 공식에 맞게 Numpy에 대해 알면 PyTorch 를 이해하기 쉽다.
가장 중요한 AutoGrad와 함께 PyTorch Operations 에 대해 알아보자
1. Tensor
- 다차원 Arrays 를 표현하는 PyTorch 클래스
- Numpy의 ndarray와 동일, TensorFlow의 Tensor와도 동일
- Tensor를 생성하는 함수도 거의 동일
# Numpy - ndarray
import numpy as np
n_array = np.arange(10).reshape(2,5)
print(n_array)
print("ndim :", n_array.ndim, "shape :", n_array.shape)
# PyTorch - tensor
import torch
t_array = torch.FloatTensor(n_array)
print(t_array)
print("ndim :", t_array.ndim, "shape :", t_array.shape)
[[0 1 2 3 4]
[5 6 7 8 9]]
ndim : 2 shape : (2, 5)
tensor([[0., 1., 2., 3., 4.],
[5., 6., 7., 8., 9.]])
ndim : 2 shape : torch.Size([2, 5])
1-1. numpy like operations
기본적으로 PyTorch의 대부분의 사용법이 그대로 적용됨
data = [[3, 5, 20],[10, 5, 50],[1, 5, 10]]
x_data = torch.tensor(data)
x_data[1:]
# tensor([[10, 5, 50],
# [ 1, 5, 10]])
x_data[:2, 1:]
# tensor([5, 20],
# [5, 50]])
x_data.flatten()
# tensor([3, 5, 20, 10, 5, 50, 1, 5, 10])
torch.ones_like(x_data)
# tensor([[1, 1, 1],
# [1, 1, 1],
# [1, 1, 1]])
x_data.numpy()
# array([[ 3, 5, 20],
# [10, 5, 50],
# [ 1, 5, 10]], dtype=int64)
x_data.shape
# torch.Size([3,3])
x_data.dtype
# torch.int64
1-2. Numpy Vs PyTorch
- PyTorch의 tensor는 GPU에 올려서 사용가능
data = [[3, 5, 20],[10, 5, 50],[1, 5, 10]]
x_data = torch.tensor(data)
x_data.device
# device(type='cpu')
# GPU를 사용할 수 있는지 확인 (M1 MAC 환경)
device = torch.device("mps") if torch.backends.mps.is_available() else "cpu"
print(f"device: {device}")
# GPU 상에서 실행 (M1 MAC 환경)
x_data = torch.tensor(data, device=device)
print(x_data)
cpu
device: mps
tensor([[ 3, 5, 20],
[10, 5, 50],
[ 1, 5, 10]], device='mps:0') <- mps:0이 출력되어야 GPU 사용한 것
2. Tensor handling
- view, squeeze, unsqueeze 등으로 tensor 조정가능
- view : reshape과 동일하게 tensor의 shape을 변환
- squeeze : 차원의 개수가 1인 차원을 삭제 (압축)
- unsqueeze : 차원의 개수가 1인 차원을 추가
2-1. view
tensor_ex = torch.rand(size=(2, 3, 2))
print(tensor_ex)
# tensor([[[0.6388, 0.0342],
# [0.2073, 0.5346],
# [0.3281, 0.5916]],
# [[0.5568, 0.2371],
# [0.0448, 0.7719],
# [0.0505, 0.3120]]])
print(tensor_ex.view([-1, 6]))
# tensor([[0.6195, 0.0416, 0.6620, 0.1878, 0.8998, 0.7728],
# [0.1560, 0.6493, 0.2845, 0.1738, 0.0421, 0.9934]])
print(tensor_ex.reshape([-1, 6]))
# tensor([[0.6195, 0.0416, 0.6620, 0.1878, 0.8998, 0.7728],
# [0.1560, 0.6493, 0.2845, 0.1738, 0.0421, 0.9934]])
2-2. squeeze & unsqueeze
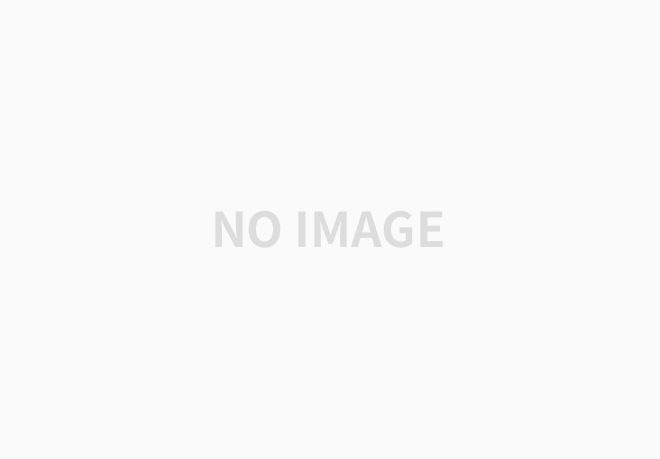
tensor_ex = torch.rand(size=(2, 1, 2))
tensor_ex.squeeze()
# tensor([[0.8510, 0.8263],
# [0.7602, 0.1309]])
tensor_ex = torch.rand(size=(2,2))
tensor_ex.unsqueeze(0).shape
# torch.Size([1, 2, 2])
tensor_ex.unsqueeze(1).shape
# torch.Size([2, 1, 2])
tensor_ex.unsqueeze(2).shape
# torch.Size([2, 2, 1])
3. Tensor Operation
- 행렬곱셈 연산은 dot이 아닌 mm 사용, 벡터내적은 dot 가능
- matmul 은 broadcasting을 지원해준다. -> 헷갈릴 수 있음. -> mm 사용
n2 = np.arange(10).reshape(5,2)
t2 = torch.FloatTensor(n2)
t1.mm(t2)
# tensor([[ 60., 70.],
# [160., 195.]])
t1.dot(t2)
# RuntimeError
t1.matmul(t2)
# tensor([[ 60., 70.],
# [160., 195.]])
4. nn.functional Module
- nn.functional 모듈을 통해 softmax, one_hot, cartesian_prod 등 많은 기능 제공
# softmax 와 one_hot 예
import torch
import torch.nn.functional as F
tensor = torch.FloatTensor([0.5, 0.7, 0.1])
h_tensor = F.softmax(tensor, dim=0) # dim 은 axis 와 같다.
h_tensor
# tensor([0.3468, 0.4224, 0.2318])
y = torch.randint(5, (10,5))
y_label = y.argmax(dim=1)
print(torch.nn.funtional.one_hot(y_label)) # one_hot 적용
tensor([[0, 1, 0, 0, 0],
[0, 1, 0, 0, 0],
[1, 0, 0, 0, 0],
[1, 0, 0, 0, 0],
[1, 0, 0, 0, 0],
[0, 0, 1, 0, 0],
[0, 0, 0, 0, 1],
[1, 0, 0, 0, 0],
[1, 0, 0, 0, 0],
[1, 0, 0, 0, 0]])
# cartesian_prod 예
import itertools
a = [1, 2, 3]
b = [4, 5]
list(itertools.product(a, b)) # 모든 경우의 수를 구해줌
tensor_a = torch.tensor(a)
tensor_b = torch.tensor(b)
print(torch.cartesian_prod(tensor_a, tensor_b)) # 모든 경우의 수를 구해줌
tensor([[1, 4],
[1, 5],
[2, 4],
[2, 5],
[3, 4],
[3, 5]])
5. AutoGrad
- PyTorch의 핵심은 자동 미분의 지원 -> backward 함수 사용
- 미분의 대상이 되는 것을 requires_grad=Ture 로 한다. (대부분 weight 값)
# Ex1
w = torch.tensor(2.0, requires_grad=True) # w = 2 이고, w에 대해 미분가능
y = w**2
z = 10*y + 25
z.backward() # .backward() 가 미분 명령어
print(w.grad)
tensor(40.)
# Ex2
a = torch.tensor([2., 3.], requires_grad=True)
b = torch.tensor([6., 4.], requires_grad=True)
Q = 3*a**3 - b**2
external_grad = torch.tensor([1., 1.])
Q.backward(gradient=external_grad)
print(a.grad)
print(b.grad)
tensor([36., 81.])
tensor([-12., -8.])
'✍🏻Language & FrameWork > Pytorch' 카테고리의 다른 글
[PyTorch] 프레임워크 소개 (0) | 2024.01.10 |
---|